Working Group Webinar Library
Webinar Library
Conventional NLP Classifiers versus Large Language Models for Risk Prediction in Clinical Care
This talk shares insights from real-world use of text-based AI at the bedside—from opioid misuse screening via EHR notes to Ambient AI for automating documentation—highlighting both opportunities and risks, as well as approaches for evaluation and improvement.
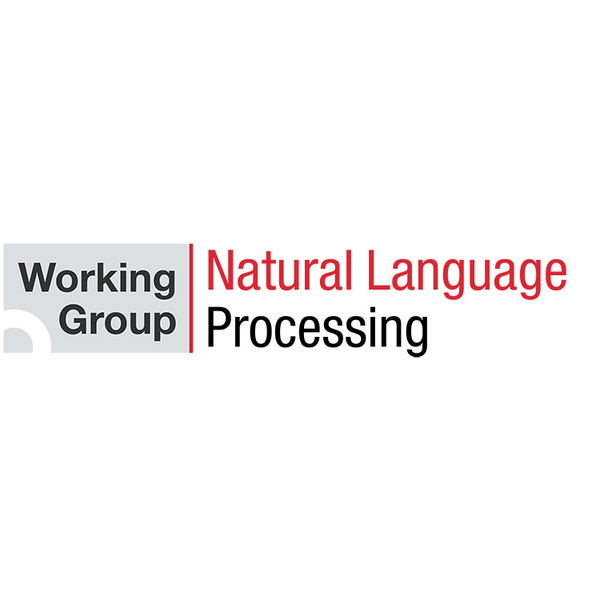
Building the LLM App That Clinicians Love: Product and AI Safety Perspectives
In this session, Dr. Peterson and Dr. Ryu will discuss their journey building an LLM application at Mayo Clinic that scaled from an idea to thousands of active users. Attention to product design and AI safety were two key factors that enabled this success and will be the focus of the session.
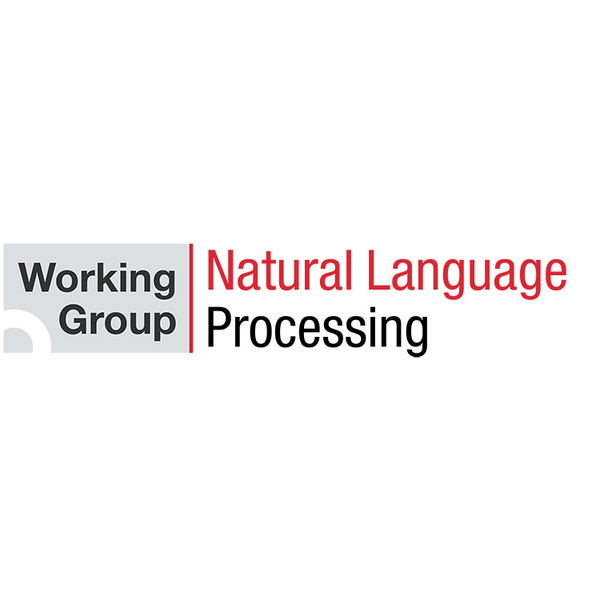
Democratizing AI for Cancer with Privacy Preserving Synthetic Data Generation for Cancer Case Identification
As part of the Department of Energy’s partnership with the National Cancer Institute, the Modeling Outcomes Using Surveillance Data and Scalable AI for Cancer (MOSSAIC) project aims to develop deep learning models to support near-real-time cancer surveillance at the population level. Through the NCI’s Surveillance, Epidemiology, and End Results (SEER) program, we deploy models for the automated coding of cancer cases across state and regional cancer registries throughout the United States.
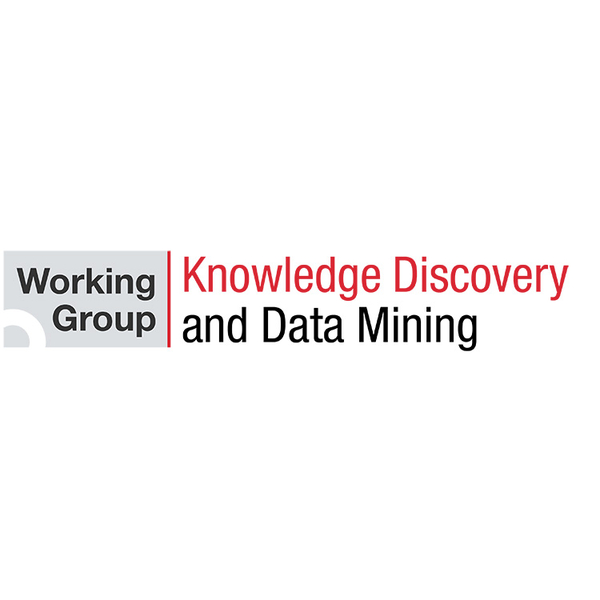
AI Scientists for Biomedical Discoveries
In this talk, James Zou, PhD explores how generative AI agents can enable drug discovery and development. He introduces the Virtual Lab—a collaborative team of AI scientist agents conducting in silico research meetings to tackle open-ended R&D projects.
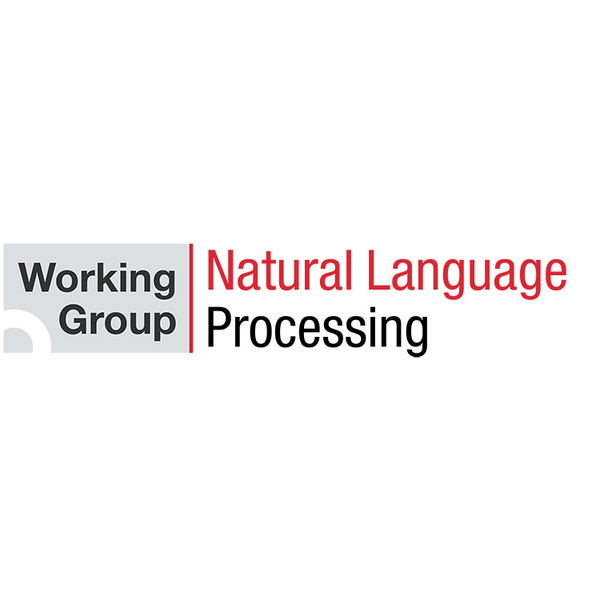
AMIA KDDM Panel: Real-World AI Implementation in Healthcare
This dynamic discussion will feature perspectives from academia, clinical practice, and industry, highlighting practical strategies for integrating AI solutions into healthcare settings. Panelists will share experiences, challenges, and lessons learned from implementation efforts, with a focus on governance, workflow integration, and impact assessment.
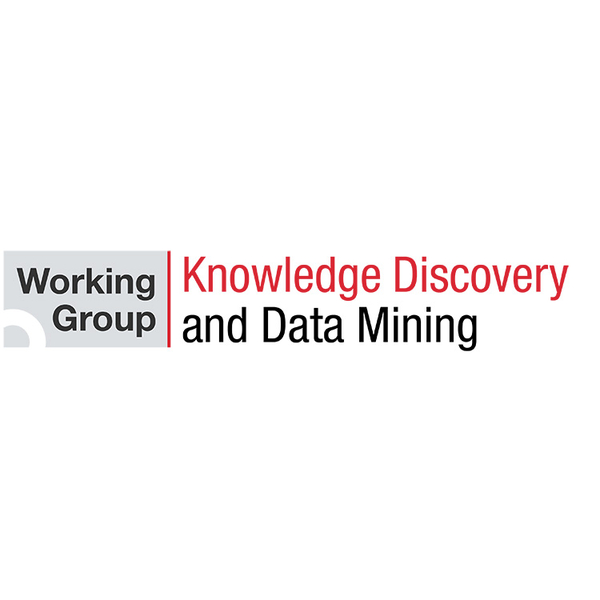