Working Group Webinar Library
Webinar Library
Clinical Research Informatics
Population Health and Pandemic Response: A New Frontier for National Statistical Institutes Using Large-scale Linked Data Assets
In this session, Emma Rourke, Director of Health Analysis and Pandemic Insights at ONS, outlines how the organization leveraged large-scale linked administrative data assets alongside ambitious survey data collection, including wide scale biological sampling, to offer unique evidence and insights that have informed the UK's pandemic response. Emma will detail how ONS stood-up the gold-standard longitudinal Coronavirus Infection Survey (CIS) in April 2020, offering trusted regular estimates of Covid-19 infection and immunity across all four nations of the UK.
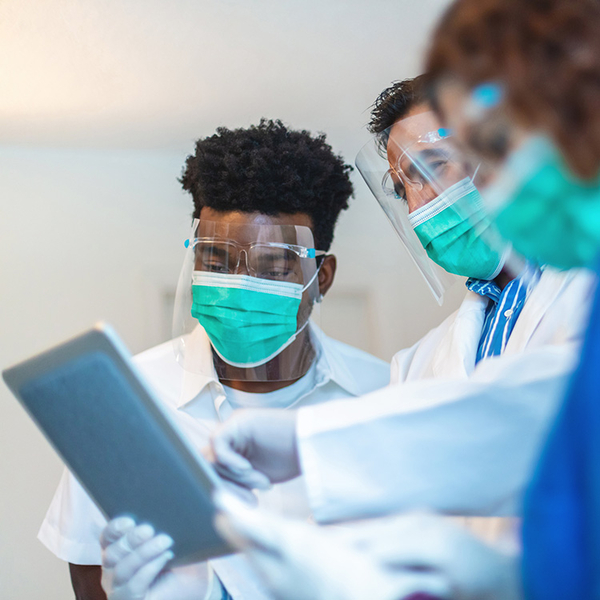
Clinical Informatics
Output and Next Steps from the 25x5 Symposium to Reduce Documentation Burden on US Clinicians by 75% by 2025
An overview of the 25 by 5: Symposiumo Reduce Documentation Burden on US Clinicians by 75% or less by 2025 from Sarah Rosetti, PhD, RN and Trent Rosenbloom, MD, MPH, FACMI, FAMIA.
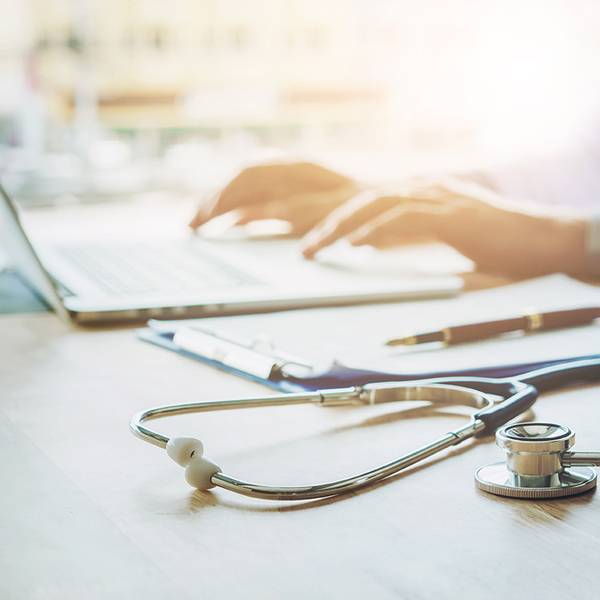
Clinical Informatics
Data Consult Service
Anna Ostropolets, MD, PhD Candidate presents "Data Consult Service Generating New Clinical Evidence at the Point of Care.
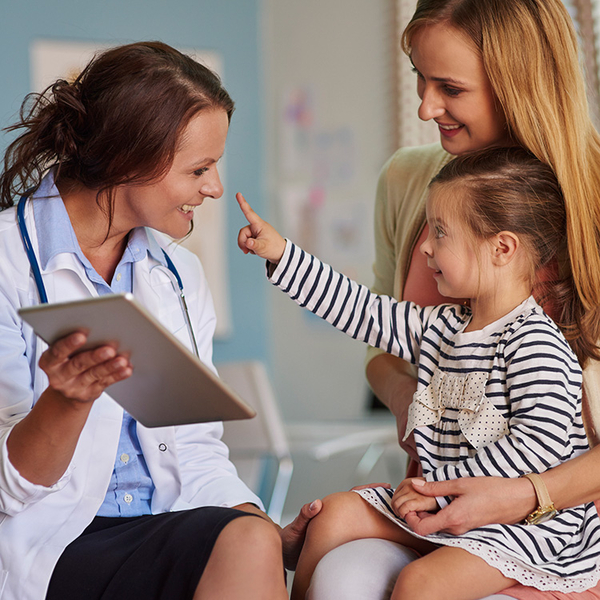
Clinical Research Informatics
From Clinical Language Representation to Patient Representation using Electronic Health Records
The widespread adoption of Electronic Health Records (EHRs) has enabled the use of clinical data for clinical research and practice. Since a significant portion of relevant patient information is embedded in clinical narratives, natural language processing (NLP) techniques such as information extraction have become critical for using EHRs in clinical research. Meanwhile, encoding entire EHR data in a patient representation has shown promising results in predictive modeling, and could help clinical decision making and facilitate translational research. This talk will feature clinical NLP methodologies and applications, and discuss why learning better representations from EHRs is crucial for clinical and translational research.
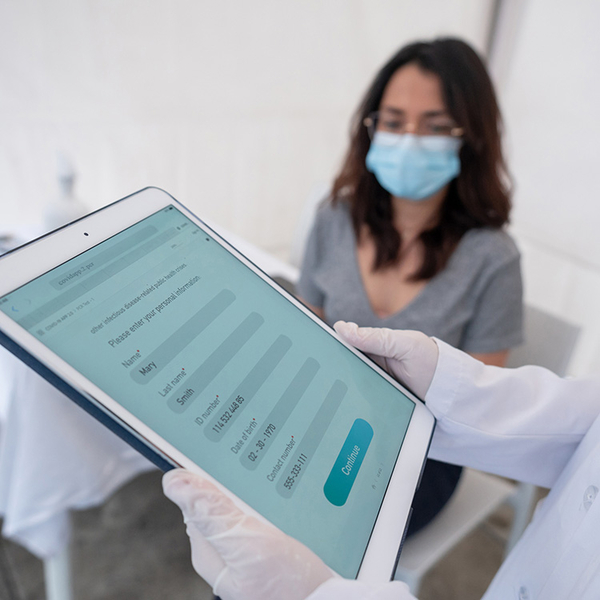
A Fireside Chat with AMIA's CEO and NIWG
Nursing Informatics Working Group leaders Dr. Judy Murphy and Dr. Karen Dunn-Lopez sat down with AMIA CEO Tanya Tolpegin and AMIA Board Member Susie Hull to discuss how NIWG can have the biggest impact on the future of nursing.
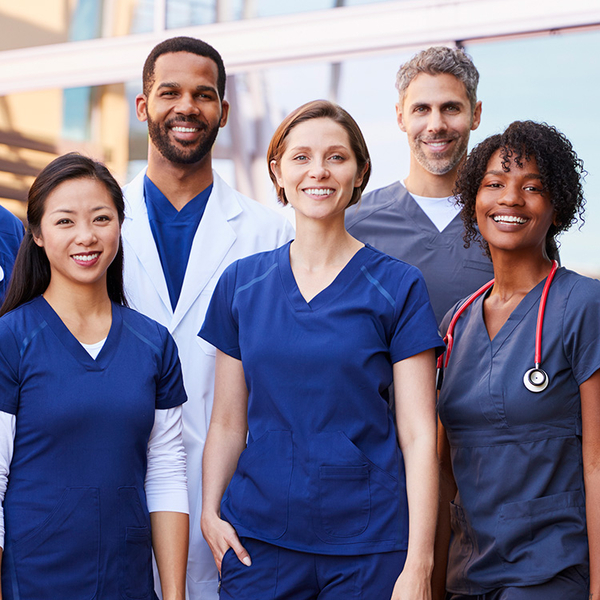