For Your Informatics (FYI) Podcast
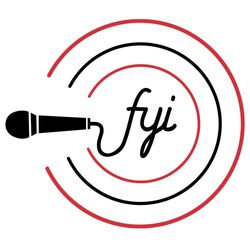
This study aims to evaluate the impact of using a large language model (LLM) for generating draft responses to patient messages in the electronic health record (EHR) system on clinicians and support staff workload and efficiency.
Author(s): Proctor, Stephon N, Lawton, Greg, Sinha, Shikha
DOI: 10.1055/a-2576-0579
Large language models (LLMs) have shown potential in biomedical applications, leading to efforts to fine-tune them on domain-specific data. However, the effectiveness of this approach remains unclear. This study aims to critically evaluate the performance of biomedically fine-tuned LLMs against their general-purpose counterparts across a range of clinical tasks.
Author(s): Dorfner, Felix J, Dada, Amin, Busch, Felix, Makowski, Marcus R, Han, Tianyu, Truhn, Daniel, Kleesiek, Jens, Sushil, Madhumita, Adams, Lisa C, Bressem, Keno K
DOI: 10.1093/jamia/ocaf045
Interruptive alerts in clinical decision support (CDS) systems are intended to guide clinicians in making informed decisions and adhering to best practices. However, these alerts can often become a source of frustration, contributing to alert fatigue and clinician burnout. Traditionally, alert burden is often assessed by evaluating total firing counts, which can overlook the true impact of highly interruptive workflows. This study demonstrates how an alert burden metric was used [...]
Author(s): Clarke, Tatyan, Kotarski, Tyler, Tobias, Marc
DOI: 10.1055/a-2573-8067
To assess the capacity of a bespoke artificial intelligence (AI) process to help medical writers efficiently generate quality plain language summary abstracts (PLSAs).
Author(s): McMinn, David, Grant, Tom, DeFord-Watts, Laura, Porkess, Veronica, Lens, Margarita, Rapier, Christopher, Joe, Wilson Q, Becker, Timothy A, Bender, Walter
DOI: 10.1093/jamiaopen/ooaf023
This work aims to develop a methodology for transforming Health Level 7 (HL7) Clinical Document Architecture (CDA) documents into the Observational Medical Outcomes Partnership (OMOP) Common Data Model (CDM). The described method seeks to improve the Extract, Transform, Load (ETL) design process by using HL7 CDA Template definitions and the CDA Refined Message Information Model (CDA R-MIM).
Author(s): Katsch, Florian, Hussein, Rada, Stamm, Tanja, Duftschmid, Georg
DOI: 10.1093/jamiaopen/ooaf022
Degenerative rotator cuff tears (DCTs) are the leading cause of shoulder pain, affecting 30%-50% of individuals over 50. Current phenotyping strategies for DCT use heterogeneous combinations of procedural and diagnostic codes and are concerning for misclassification. The objective of this study was to create standardized phenotypic algorithms to classify DCT status across electronic health record (EHR) systems.
Author(s): Herzberg, Simone D, Garduno-Rapp, Nelly-Estefanie, Ong, Henry H, Gangireddy, Srushti, Chandrashekar, Anoop S, Wei, Wei-Qi, LeClere, Lance E, Wen, Wanqing, Hartmann, Katherine E, Jain, Nitin B, Giri, Ayush
DOI: 10.1093/jamiaopen/ooaf014
Despite the recent adoption of large language models (LLMs) for biomedical information extraction (IE), challenges in prompt engineering and algorithms persist, with no dedicated software available. To address this, we developed LLM-IE: a Python package for building complete IE pipelines.
Author(s): Hsu, Enshuo, Roberts, Kirk
DOI: 10.1093/jamiaopen/ooaf012
Sepsis recognition among infants in the Neonatal Intensive Care Unit (NICU) is challenging and delays in recognition can result in devastating consequences. Although predictive models may improve sepsis outcomes, clinical adoption has been limited. Our focus was to align model behavior with clinician information needs by developing a machine learning (ML) pipeline with two components: (1) a model to predict baseline sepsis risk and (2) a model to detect evolving [...]
Author(s): Cao, Lusha, Masino, Aaron J, Harris, Mary Catherine, Ungar, Lyle H, Shaeffer, Gerald, Fidel, Alexander, McLaurin, Elease, Srinivasan, Lakshmi, Karavite, Dean J, Grundmeier, Robert W
DOI: 10.1093/jamiaopen/ooaf015
Machine learning (ML) algorithms are promising tools for managing anemia in hemodialysis (HD) patients. However, their efficacy in predicting erythropoiesis-stimulating agents (ESAs) doses remains uncertain. This study aimed to evaluate the effectiveness of a contemporary artificial intelligence (AI) model in prescribing ESA doses compared to physicians for HD patients.
Author(s): Lim, Lee-Moay, Lin, Ming-Yen, Hsu, Chan, Ku, Chantung, Chen, Yi-Pei, Kang, Yihuang, Chiu, Yi-Wen
DOI: 10.1093/jamiaopen/ooaf020